
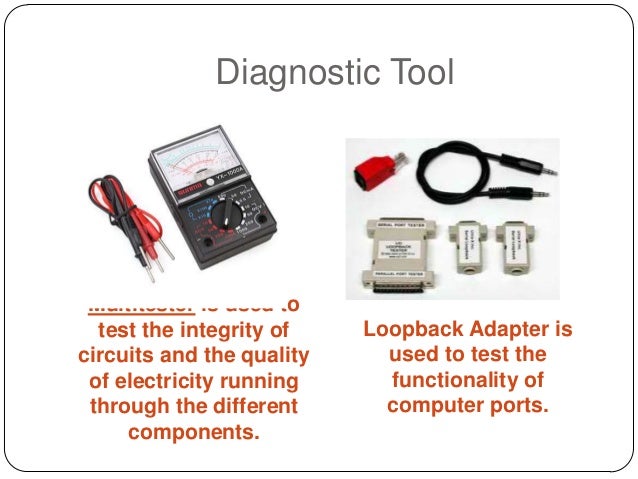
Instead, the anomaly localization assigns to each pixel, or image patch, a score used to generate a region of interest (ROI), i.e., a bounding box, around each anomaly. In more detail, the anomaly detection task is a binary classification between two classes, i.e., normal and abnormal. Generally, such tasks are performed by exploiting vision-based methods, i.e., optical analysis, processing images acquired through visual sensors to look for heterogeneous or unexpected patterns in the input images that differ from what is considered the normality. Indeed, a timely conduct of quality inspections in the production process can avoid wasting labor and material resources. Therefore, two key factors for the optimization of industrial inspections are the automatic detection and localization of structural faults, to ensure high-quality products and economic benefits for the factory. In industry, anomalies can be associated with defects that can occur during the creation, manufacturing, or transportation of products. A system capable of performing autonomously such tasks can effectively be applied to increase the features of a wide range of application fields, such as area monitoring (Ata-Ur-Rehman et al., 2021, Avola. Among the others, anomaly detection and localization are some of the most important and useful application-driven tasks that aim to, firstly, detect data samples that show different characteristics relative to a predefined notion of normality and secondly, once identified, localize the regions presenting an anomaly (Ganokratanaa et al., 2020). In the last few years, automatic vision-based systems are becoming very useful support tools for multimedia content-based tasks, e.g., activity recognition (Avola et al., 2020a, Nath and Hazarika, 2021), person re-identification (Avola et al., 2019b, Wang et al., 2021), or human behavior understanding (Avola et al., 2020, Borges et al., 2013). What is more, measuring the time required to detect and localize the defects, the trained network is run on the RPi4B as an embedded system to simulate a practical industrial setting with limited computational resources, demonstrating the applicability of the presented method in real scenarios. Ablation studies varying the number of layers are performed on all the architecture components, founding that the presented two-branch network is consistently robust among all classes achieving remarkable results, i.e., 98% for AUROC and 94% for SSIM. The effectiveness of the proposed method is evaluated on the MVTec-AD industrial benchmark comprising different object and texture categories, considering the common state-of-the-art AUROC and SSIM metrics for the evaluation of anomaly detection and localization, respectively. In both cases, the underlying strategy lies in a semi-supervised setting observing only defect-free product images, enabling the learning of the correct product structure that can be used to identify every kind of defect independently from position, color, or shape. To this end, this paper introduces a two-branch neural network architecture that comprises detector and localizer components, where the former identifies the presence of defects, while the latter defines the region of interest for each defective area detected in the product structure.
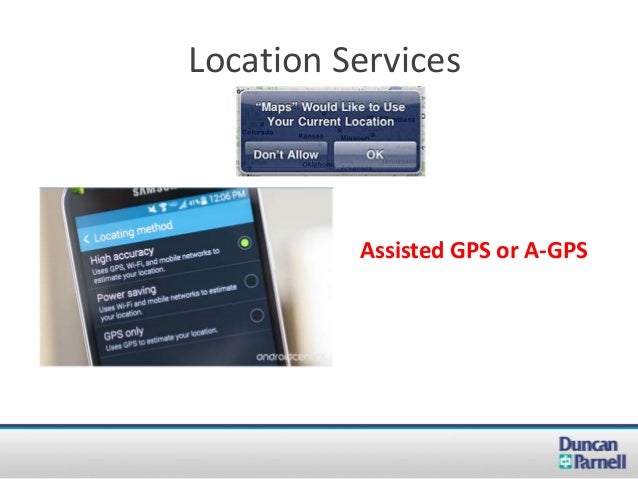
For industrial inspections, the automatic detection and localization of defective parts from product images ensure quality while avoiding waste of labor and materials. In recent years, artificial intelligence has been applied in the industry to automate various vision-based applications, such as monitoring structural defects in manufactured products.
